As the current world depends on the use of data, it is crucial to acknowledge the significance of data quality. Data quality means the suitability, reliability, and comparability of data on which many organizations depend for minimizing risks, maximizing gains, and generally making important decisions. If sufficient quality data is not acquired, there is likelihood that managers are likely to make wrong decisions that are costly for the business.In the current world with a constantly changing technological realm, companies have found themselves in possession of huge amounts of data.
Table of Contents
However, if the value and quality of the data being gathered is not given primary attention and importance, that data turns out to be a. In the current world with a constantly changing technological realm, companies have found themselves in possession of huge amounts of data.
In this blog, you will learn what data quality is and why it is important for your organization. This article will explain the importance of data quality to anyone, from the data scientist to the business analyst to the company’s executive. With respect to the management of data, it is crucial if you are going to win, gain higher results and achieve sustainable performance.
What is Data Quality?
Before going deeper into the discussion of data quality dimensions it will be pertinent to explain what data quality actually is. Here are two key definitions:
- Data that accurately reflects the real world can be considered high quality.
- Data that is fit for a specific purpose or use-case is of good quality.
Data quality is defined by one’s ability to accomplish what is desired with the data that is available. The above findings imply that usability is good if the data in it is accurate, consistent and relevant and is updated frequently. When the data is filled with mistakes, or the info is old, relying on it to make decisions is quite challenging. It’s like cooking – when the ingredients are good everything is great, but if they are not then it’s the other way around.
High-quality data shares a set of essential characteristics, including:
- Accuracy: The collected information is accurate and credible.
- Completeness: All parameters with relevant information are represented.
- Consistency: The data is the same for different sources or across different platforms.
All of these dimensions are rather significant in how well the data may be used to make the successive decisions. Lack of nugget 3 knowledge of data quality can be a real problem for organizations giving them difficulties in comprehending their data and using them for their goals.
Hevo ensures that your data transfers are secure and reliable, with no data loss. Experience end-to-end encryption for complete peace of mind.
- Zero Data Loss: Safeguard your data from source to destination.
- Complete Data Security: Enjoy encrypted transfers to protect your sensitive information.
- Efficient ETL/ELT: Process data in real-time without compromising security or performance.
Trust Hevo to handle your ETL/ELT needs with unparalleled efficiency and security.
Get Started with Hevo for FreeWhy is Data Quality so important?
Data quality is a key factor that enables informed decisions and operations, allows avoiding additional costs, and builds trust with customers. Precise or high quality data can then effectively reduce risks, reduce expenditure, and enhance positive organizational relationships with the target audience.
Making informed decisions starts with high-quality data: When the data collected is credible, leadership is in a better position to make informed decisions and hence minimize the risks, and enhance on positive returns.
Cost Efficiency: Bad data results in negative consequences, while with good and clean data, unnecessary correction of errors and wastage of money are avoided.
Customer trust is another big reason data quality matters: The audience wants correct information and it is always helpful when providing it – helps to build up the brand and attract more people. In a business environment where it is sometimes difficult to believe, making sure that numbers are accurate can help make the difference.
It is easier to enhance data quality and make the right decision, customers are always happy, and costs are reduced by doing business with better data quality.
What are the Six Elements of Data Quality?
The introduction to the six dimensions of data quality is crucial to creating sound data-driven organizations. Each component has a huge responsibility of ensuring that data is accurate, consistent, and reliable with a view of improving organizational decision-making and operations. These are basic components of any organization seeking to harness data in its operations.
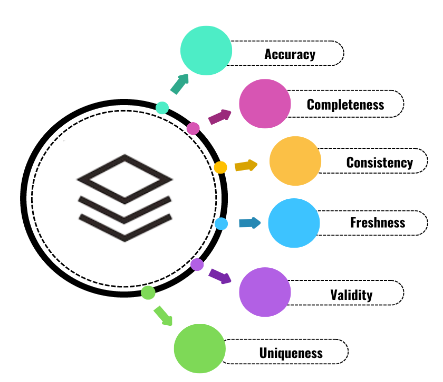
- Accuracy: This relates to data accuracy, which means that the data entered is correct. Accuracy refers to the level where the data collected is in accordance with the items depicted in the data rather than reflections of real life. For instance, when inputting a customer’s details, they must be correct or else you will lose their contact.
- Completeness: The type, quality, and quantity of facts you have at your disposal will likely affect the conclusion you draw, therefore this is absolutely accurate. Completeness attests to the presence of all necessary data in the systems. Consider a client profile without an email address; this would be somewhat prohibitive of contact.
- Consistency: Using tables and reports, information must be uniform over the different reports or dataset. Where one report indicates a customer’s purchase history, another has some problems, thus putting the customers in this confusion. It is useful for keeping things clear, which is already a major advantage.
- Freshness: Another essential requirement is that information should be updated. That is, timeliness of information guarantees that decisions are made using relevant information. For instance, promotions with season dating based on old data are a bad idea and will not help to make sales.
- Uniqueness: Since each entity in unique data appears once, this kind of data reduces redundancies, inconsistencies, and errors. I was thinking that when the same customer is in the two lists, there is confusion that ensues making work complicated.
- Validity: This element confirms compliance or otherwise of the data with certain business rules or constraints. Cohort variables are acceptable and applicable based on certain criteria like format and/or range of data encountered.
Best Practices for Ensuring Data Quality
In the modern context of constantly increasing amounts of information, the requirement to maintain high data quality remains crucial for Sustainable Competitive Advantage. Here are some key best practices that can help ensure your organization’s data remains accurate, consistent, and reliable.
- Establish Clear Data Governance:
Develop a clear data accountability map that defines responsibilities for data ownership across the entire organization. This means that there are specific directions in the process to follow to maintain high quality of data throughout the process. A strong data governance framework ensures that these responsibilities are aligned with policies, procedures, and standards to maintain data integrity, security, and compliance.
- Define Data Quality Standards:
Provide clear guidelines as to what high-quality data should ideally look like in terms of accurately, completeness, consistency and timeliness. Once expectations are set, it becomes simple for teams to identify what could be wrong and correct data.
- Conduct Regular Data Audits:
This maintains the credibility of the data by earmarking certain checks to be run time to time for example, data audits to discover missing values, duplicated or outdated results. Audits should be performed routinely so that your data isn’t out of control and small mistakes are not compounded.
To Learn More look at How to Improve Data Quality.
What are the Benefits of Data Quality?
Currently, everyone who wants their organization to succeed needs quality data. Here are some key benefits of prioritizing data quality:
- Enhanced Operational Efficiency: When your data is of high quality, it cuts across all aspects of the organization making working easier. It helps teams to concentrate on what they are meant to be doing instead of spending hours attempting to eliminate mistakes or sift through unadmirable data. The former enhances production while the latter creates a better team spirit in work places.
- Better Analytics and Insights: Proper data is critical in analysis in order to produce meaningful results. Data is useful when it is accurate, as it results in the provision of recommendations for the business. This results in the remedy of deficiencies in usable information available to decision-makers capable of aiding decision makers in recognising opportunities, challenges and overall growth.
- Regulatory Compliance: Most industries are bound by many rules and regulations with the compliance of which accurate record keeping is mandatory. High-quality data makes an organization meet compliance requirements thereby cutting the risk of imposing fines and penalties.
- Enhanced Collaboration: When data is stable and accurate, cross functional teams are enhanced as employees within the organizations collaborate. Integration fortification, often referred to as collaboration enhancement results from timely and proper communications that facilitate in attaining a mutual understanding of goals for business improvement endeavors.
Conclusion
In today’s data-driven environment, understanding data quality is not just beneficial—it’s essential. High-quality data not only informs better decisions but also saves costs and fosters trust. Embracing the importance of data quality can transform how organizations operate and thrive. As we have entered the data driven era, it is imperative that business organizations understand these data qualities. Even though proper utilization of big data relies on availability of extensive data, it is crucial to assure that data is credible and useful. The quality of data acquisition provides the necessary effect on the improvement of decision-making processes, optimization of operations, and maintaining trust with the consumer.
By addressing specific attributes, such as accuracy, completeness, consistency, timeliness, specificity, and relevancy, organizations can open the door to valuable insights and square off with problems instead of trying to avoid them. The best data reduces the compliance risks, and it allows the teams to work better, where everyone will be able to solve their problems.
Hevo helps you maintain high data quality standards to make data-driven decisions based on reliable and accurate information. Sign up for a 14-day free trial today.
FAQ
What does data quality mean?
Data quality is defined as the extent to which a set of data fulfills the purpose that is intended to be served. It contains different attributes such as accuracy, comprehensiveness, uniformity, currency, Illegitimacy, and legitimacy.
What are the 5 elements of data quality?
The five key elements of data quality include:
Accuracy: Data should be correct and reflect the real-world situation.
Completeness: All necessary data points must be included.
Consistency: Data should be uniform across different sources or systems.
Freshness: Data must be up-to-date to ensure timely decisions.
Validity: Data should comply with relevant business rules and constraints.
What does poor data quality mean?
Data quality is defined as data that is incorrect, incomplete, conflicting, out of date or irrelevant. This kind of information can result in wrong assumptions, wrong approaches, time, and money being invested in wrong areas, and customer distrust.
How do you measure data quality?
There are general measures used to measure data quality, these include the accuracy, completeness, consistency, timeliness and validity of data. Currently used forms are data profiling, validation, and audit procedures to determine inconsistencies and opportunities for development.