Picture this: you’re in an agitated rush to the hospital due to a serious allergic reaction, and the doctor quickly reviews your electronic health record. Unfortunately, the allergy information is not present resulting in the doctor to give a medication that might cause a more severe reaction. Terrifying. This clearly shows the significant impact that inadequate data quality can have on health care results. High-quality data goes beyond just improving efficiency. It plays an essential role in protecting lives.
Table of Contents
A report from the World Health Organisation (WHO) reveals that almost 40% of healthcare expenditures are wasted due to poor healthcare with insufficient and low quality data being a major contributing factor. Healthcare facilities around the globe are embracing a more analytical approach, utilising digital platforms to manage, analyse and share essential patient data. The challenge is to ensure that this data is accurate, timely, comprehensive, and easily accessible. When data is inaccurate or incomplete, it can result in incorrect diagnoses, postponed treatments and negative consequences for patients. In this blog, we will examine the significance of data quality in healthcare, methods for its assessment and strategies organisations can do to strengthen it.
Introduction to Data Quality in Healthcare
In healthcare, “data quality” means how correct, dependable, and up-to-date the information is across different platforms, such as Electronic Health Records (EHRs), patient polls and claims data. What does “data quality” really mean when we talk about healthcare? This involves making sure that all information is accurate and present, including a patient’s diagnosis, medication list and examination results.
Healthcare data must be correct. Medical professionals use this information to make choices that could save lives and one mistake could have terrible results. Furthermore, precise and prompt data is essential in various areas of healthcare, including policy development, research initiatives and the management of population health. In the realm of healthcare, the absence of quality data can lead to significant difficulties, even for the most sophisticated systems.
Data Sources for Health Care Quality Measures
Data is gathered from a variety of sources to assess the quality of healthcare, with each source fulfilling its specific role. Let us explore some of the most frequently used data sources:
- Electronic Health Records (EHRs): EHRs are probably the most important source of healthcare data. These digital records offer a thorough overview of a patient’s medical background, encompassing diagnoses, medications, allergies, lab results and imaging studies. However, it is important to know that the accuracy of the data in EHRs relies heavily on the input from healthcare providers and their staff.
- Claims Data: This information is sourced from insurance claims filed by healthcare providers. This provides a glimpse into the various treatments that patients are undergoing and their effectiveness. Data related to claims is frequently utilised to track patient outcomes, maintain checking on healthcare expenses, and evaluate the effectiveness of care offered.
- Patient surveys: Self-reported data from patients is another useful resource for assessing healthcare quality. These surveys offer insights into how patients feel regarding their care, overall quality of life and satisfaction levels with treatments. Since the nature of patient-reported data is subjective, it may pose challenges regarding the data accuracy.
- Laboratory Reports: Lab tests and imaging studies such as MRIs, CT scans, and X-rays are data sources for accurate diagnosis. These reports offer insights into the patient’s health and allow professionals to make better decisions in their patient’s treatments.
- Specialized Registries: Long-term data regarding specific diseases, like diabetes or cancer, are gathered and tracked by registries that are dedicated to a particular disease. These registries offer valuable perspectives on how diseases evolve and how treatments perform as time goes on.
Importance of Data Quality in Healthcare
Each of these sources is essential for holding all aspects of healthcare quality, although their effectiveness is largely dependent on the quality of the data they offer.
Data quality is vital in the healthcare sector. Poor data quality can result in unfavourable patient outcomes, financial losses, and operational inefficiencies throughout healthcare organisations. When data quality meets a high level, the benefits are enormous, including improved patient outcomes, more efficiency in healthcare delivery, and lower costs.
Consider a study from 2024. A significant hospital system introduced data validation processes in its emergency departments. The hospital reduced diagnostic errors by ensuring that patient data were consistently updated and correct. This resulted in faster and more accurate treatments, greatly increasing patient outcomes in critical care conditions.
Inaccurate data can result in serious repercussions. Imagine a scenario in a hospital where the use of outdated information resulted in patients receiving medications to which they had allergies. A patient may face a life-threatening situation if their penicillin allergy is not properly recorded in the hospital’s electronic health record system. If the data was correct, the situation might have been avoided.
Key Metrics for Measuring Data Quality in Healthcare
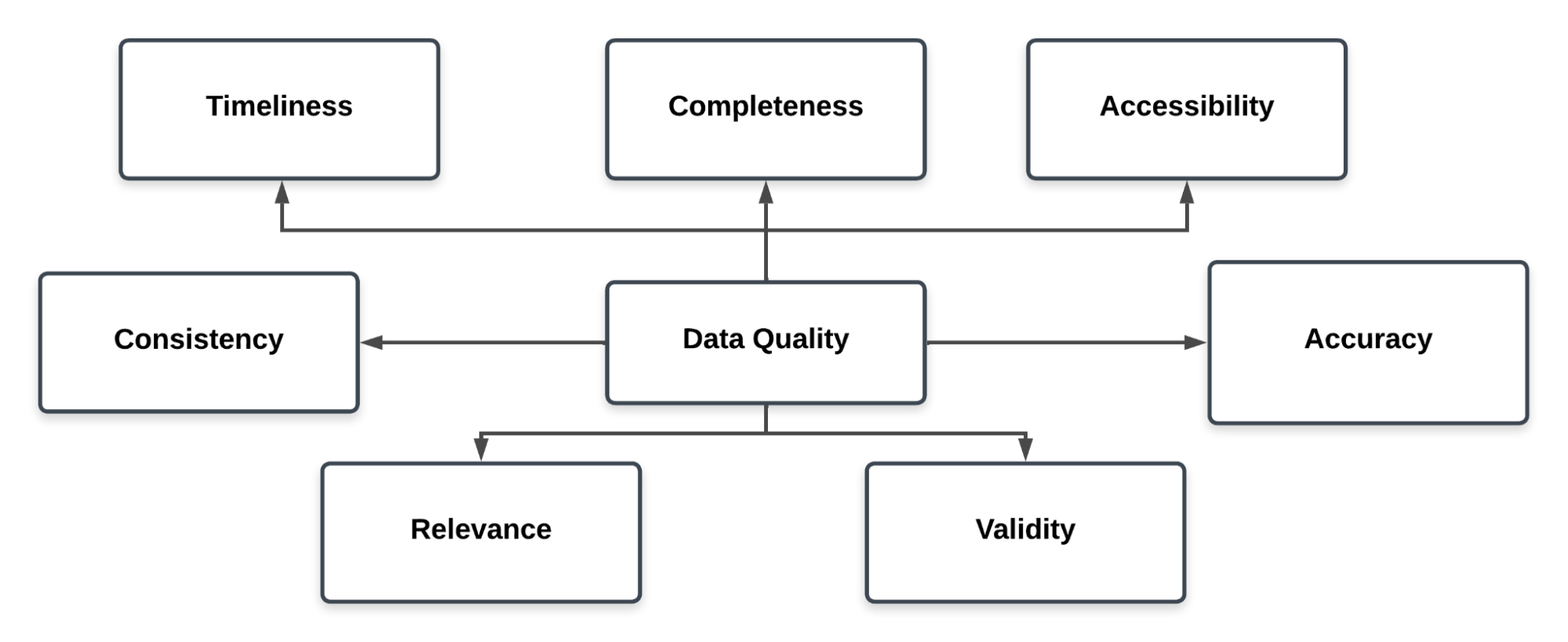
Healthcare organisations rely on a variety of key indicators to assess data quality and ensure that the information is accurate and useful.
- Accuracy: Accuracy stands out as the most important aspect of data quality. The data should be correct including a patient’s diagnosis, medication list and lab results. Inaccurate data can lead to inaccurate diagnosis and bad treatments which may become harmful. Hence, auditing and validating data should be a compulsory process in data accuracy.
- Completeness: Missing data is a big challenge in the healthcare sector. When a patient’s medical history lacks completeness, healthcare professionals might not possess all the necessary information to make favourable decisions. For making beneficial decisions, you need all the information you can get.
- Timeliness: Timing is everything. When patients experience delays in treatment, it can lead to more severe consequences for their health. Data must be up-to-date and accessible. In a intensive care units, treatment delays caused by outdated information can make it much less likely for a patient to get better after being diagnosed.
- Consistency: Data that is constant across several platforms and systems is known as data consistency. When a patient’s blood pressure appears as 120/80 in one system, it can’t show up as 130/90 in another. When people get incorrect information, it can lead to misunderstandings and mistakes in how they treat patients.
- Accessibility: The sensitive data that healthcare professionals require must be easily and quickly accessible to authorised professionals. If a doctor is unable to get a patient’s information quickly, treatment may have to postpone or could be given to the wrong person.
- Validity: Data validity makes sure that the data collected is correct and follows standard medical practice. For example, lab data must be given in the right units and diagnoses have to be written according to established standards like the International Classification of Diseases (ICD).
- Relevance: Some data is not relevant at all. Relevance guarantees that the information gathered has a significant and valuable function. Collecting detailed information about a patient’s dietary habits can be crucial for a diabetic patient while it may be irrelevant for someone receiving treatment for a broken bone.
Steps to Ensure Data Quality in Healthcare
In healthcare, keeping accurate data is not easy, but it is essential. Here are some important things healthcare organisations can do to keep and improve the quality of their data:
- Data Validation and Regular Checks
Adding validation checks at the point of entry is one of the best ways to make sure the quality of the data. Checking healthcare data on a regular basis can help fix mistakes before they hurt patient care. As an example, a hospital could check its patients’ records once a month to make sure that all allergies are recorded properly and are up to date.
- Training Healthcare Staff
One of the main reasons for poor data quality in healthcare is human error. Healthcare organisations can greatly lower the chance of mistakes by training their staff comprehensive methods on how to enter and manage data.
- Data Governance
Data governance involves establishing clear rules and policies on how data is collected and used. We have to decide who has access to certain kinds of data and also see that all regulatory rules are followed. It is important to have a strong data control system to keep healthcare data safe.
- Real-Time Monitoring
Monitoring tools that work in real time can find errors in data as they occur and also continously track them. These systems can track metrics such as accuracy and relevance and alert when something goes bad. Monitoring in real time can help keep small data concerns from getting worse.
Common Data Quality Issues and Challenges in Healthcare
Despite their best efforts, healthcare companies continue to face significant data quality challenges. In most circumstances, the following are the issues that arise:
- Data Silos
In many cases, healthcare data is kept in different systems that don’t communicate with each other. This makes data silos. This could indicate that patient records are incomplete and there are discrepancies between departments. When data is maintained in multiple locations, it is difficult to have a complete picture of a patient’s health, leading to gaps in healthcare.
- Inconsistent Data Entry
Data may not be consistent because healthcare professionals may enter the same information in different ways. Inconsistent practices make it difficult to compare data across different departments.
- Human Error
Data mistakes happen all the time. A simple mistake or omission can cause statistics to be wrong, which can have very bad effects on patient care. For example, if one single point is missing from a medicine dosage, the patient could get ten times the dose that was supposed to be given. It is very critical to look for human errors.
- Outdated Tech
A lot of healthcare organisations are still using old tech like paper notes or legacy systems that can’t handle the data needs of today. Mostly these systems don’t have the tools that ensure that the data is consistent.
- Duplicate Records
It is usual to keep numerous copies of a patient’s record. When a patient is enrolled in many systems, it might be difficult to maintain track of them.
Data Quality Tools in Healthcare
Common tools include Electronic Health Records (EHR) systems, which centralise patient data and automate data entry. Data validation software detects mistakes and inconsistencies. Master Data Management (MDM) solutions standardise data across systems, whereas Data Quality Dashboards enable real-time monitoring of metrics. Tools like predictive analytics platforms rely on clean data for forecasting health outcomes. These tools make care better by reducing errors, speeding up processes, and allowing for more precise interventions. This improves patient safety and the efficiency of healthcare as a whole.
Conclusion
Data quality is essential for providing effective healthcare. High-quality data empowers the medical industry to make better decisions that ultimately benefit their patients. By working on key factors such as correctness, timeliness, and accessibility, medical institutions may increase the reliability of their data and reduce the possibility of errors in the data.
It’s not just about saving time and money to invest in data quality tools and processes; it’s also about saving lives. Healthcare organisations must put data quality first in order to give their patients the best care possible. This can be done through real-time monitoring, automated data validation, or staff training. Better data leads to better health outcomes for everyone.
Frequently Asked Questions
1. What are data quality measures?
Data quality measures are the basic standards used to evaluate how accurate, complete and reliable data is. In healthcare, these data quality metrics are used to ensure that professionals have the necessary information to make decisions.
2. What are the three main measures of medical care quality?
The three primary metrics for evaluating the quality of medical care are structure (the organisation of a healthcare system), process (the manner in which healthcare is administered), and outcomes (the outcomes of the healthcare provided).
3. What are examples of quality measures in healthcare?
There are a great number of examples. Scores on patient satisfaction, rates of infection, rates of hospital readmission, and rates of medication administration that are completely accurate are some examples.
4. What are the 7 C’s of data quality?
The 7 Big C’s of data quality are Completeness, Consistency, Conformity, Correctness, Credibility, Currentness, and Contextualization.